Researchers from Children’s Hospital of Philadelphia (CHOP) have developed a new tool to help analyze data collected from spatial transcriptomics technologies (SRTs), which simultaneously profile gene expression and spatial location information in tissues to pinpoint which cells in which layers of tissue may drive various diseases. The findings, published recently in the journal Nature Methods, enable researchers to better identify and understand new therapeutic targets for optimized treatment strategies.
SRTs are powerful tools with incredible versatility. Some methods profile tens of thousands of genes on thousands of locations on a tissue, while others measure hundreds of genes at a single-cell level. However, with so much data, it can be challenging to identify which cells are expressing which genes and how these might cause diseases. For example, in the brain it’s difficult for researchers to distinguish which genes are active in the different types of cells and neurons in its complex layers.
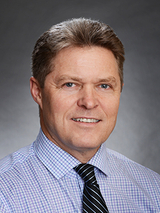
“While the data being generated by SRTs is important, by itself it is difficult to understand the big picture of how this information can be translated in a way that helps drive discovery,” said senior study author Hakon Hakonarson, MD, PhD, director of the Center for Applied Genomics (CAG) at CHOP. “This tool helps cluster the data to identify relationships between different data points and data types better than any other method currently available.”
To tackle this issue, researchers from the CAG at CHOP developed a new tool called spaVAE designed to handle the unique challenges of spatial gene data. Using advanced deep learning techniques, spaVAE is designed to understand patterns in the data, especially how different data points relate to each other in a three-dimensional part of the tissue. After collecting data from a variety of SRTs, spaVAE helps simplify complex data, creating visual representations of the data, combining data from different experiments, predicting gene activity in unmeasured areas of tissue, identifying differences in gene activity, and most importantly, finding genes that vary across different spatial regions.
Additionally, the researchers adapted spaVAE to handle multiple types of data, including data captured from multi-omics sources – the genome, proteome, transcriptome, metabolome, and epigenome.
“Using what we have learned from this study, we hope this approach could be used to ascertain more meaningful data from other spatial genomic technology, allowing us to investigate cell-to-cell communication and delving deeper into single cell genomics data,” said Tian Tian, PhD, a former postdoctoral fellow with the CAG laboratory at CHOP.
This study was supported by grant National Institutes of Health grant R15HG012087, grant BK20230781 from the Natural Science Foundation of Jiangsu Province, and also funded in part by an Institutional Development Fund from CHOP and by CHOP’s Endowed Chair in Genomic Research. This work used the Extreme Science and Engineering Discovery Environment (XSEDE) through the allocation CIE170034, supported by the National Science Foundation grant number ACI1548562.
Tian et al, “Dependency-aware deep generative models for multitasking analysis of spatial omics data.” Nat Methods. Online May 23, 2024. DOI: 10.1038/s41592-024-02257-y.
Researchers from Children’s Hospital of Philadelphia (CHOP) have developed a new tool to help analyze data collected from spatial transcriptomics technologies (SRTs), which simultaneously profile gene expression and spatial location information in tissues to pinpoint which cells in which layers of tissue may drive various diseases. The findings, published recently in the journal Nature Methods, enable researchers to better identify and understand new therapeutic targets for optimized treatment strategies.
SRTs are powerful tools with incredible versatility. Some methods profile tens of thousands of genes on thousands of locations on a tissue, while others measure hundreds of genes at a single-cell level. However, with so much data, it can be challenging to identify which cells are expressing which genes and how these might cause diseases. For example, in the brain it’s difficult for researchers to distinguish which genes are active in the different types of cells and neurons in its complex layers.
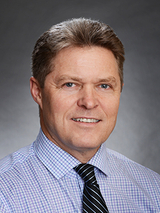
“While the data being generated by SRTs is important, by itself it is difficult to understand the big picture of how this information can be translated in a way that helps drive discovery,” said senior study author Hakon Hakonarson, MD, PhD, director of the Center for Applied Genomics (CAG) at CHOP. “This tool helps cluster the data to identify relationships between different data points and data types better than any other method currently available.”
To tackle this issue, researchers from the CAG at CHOP developed a new tool called spaVAE designed to handle the unique challenges of spatial gene data. Using advanced deep learning techniques, spaVAE is designed to understand patterns in the data, especially how different data points relate to each other in a three-dimensional part of the tissue. After collecting data from a variety of SRTs, spaVAE helps simplify complex data, creating visual representations of the data, combining data from different experiments, predicting gene activity in unmeasured areas of tissue, identifying differences in gene activity, and most importantly, finding genes that vary across different spatial regions.
Additionally, the researchers adapted spaVAE to handle multiple types of data, including data captured from multi-omics sources – the genome, proteome, transcriptome, metabolome, and epigenome.
“Using what we have learned from this study, we hope this approach could be used to ascertain more meaningful data from other spatial genomic technology, allowing us to investigate cell-to-cell communication and delving deeper into single cell genomics data,” said Tian Tian, PhD, a former postdoctoral fellow with the CAG laboratory at CHOP.
This study was supported by grant National Institutes of Health grant R15HG012087, grant BK20230781 from the Natural Science Foundation of Jiangsu Province, and also funded in part by an Institutional Development Fund from CHOP and by CHOP’s Endowed Chair in Genomic Research. This work used the Extreme Science and Engineering Discovery Environment (XSEDE) through the allocation CIE170034, supported by the National Science Foundation grant number ACI1548562.
Tian et al, “Dependency-aware deep generative models for multitasking analysis of spatial omics data.” Nat Methods. Online May 23, 2024. DOI: 10.1038/s41592-024-02257-y.
Contact us
Ben Leach