A research team at Children’s Hospital of Philadelphia (CHOP) has developed an innovative computational tool offering researchers an efficient method for detecting the different ways RNA is pieced together (spliced) when copied from DNA. Because variations in how RNA is spliced play crucial roles in many diseases, this new analytical tool will provide greater capabilities for discovering disease biomarkers and therapeutic targets, even from RNA-sequencing data sets with modest coverage.
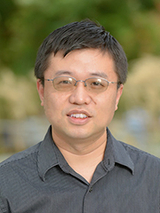
Study leader Yi Xing, PhD, Director of the Center for Computational and Genomic Medicine at CHOP, and first authors and PhD students Zijun Zhang and Zhicheng Pan report on their DARTS framework this week in Nature Methods. DARTS (Deep-learning Augmented RNA-seq analysis of Transcript Splicing) uses deep-learning based predictions to harness the wealth of information available in public datasets of RNA sequencing (RNA-seq), thus allowing for new insights into alternative splicing.
“The conceptual innovation of DARTS is it provides a bridge from big data in the public domain to smaller data sets in focused studies with individual investigators,” said Xing. “DARTS offers the ability to transform massive amounts of public RNA-seq data into a knowledge base, represented as a deep neural network, of how splicing is regulated. Using this computational framework, we can push that into any individual lab. This could be really useful and increase the efficiency of the experiment and enable new discoveries. With just 20 or 30 million RNA-seq reads, you can make educated guesses and inferences on things you were never able to see in the past.”
Xing has a long-standing research focus on alternative splicing — the process by which information in DNA of a single gene is pieced together in different ways to generate different messenger RNA and protein products after gene transcription. Genes each generate an average of 10 or more such products, and sometimes as many as 38,000. Those variations in alternative splicing may cause disease, modify disease risk, or make a disease milder or worse.
Massively parallel RNA sequencing is now the standard technology researchers use to investigate alternative splicing. However, to accurately measure alternative splicing, the RNA sequencing experiments have to go very deep. The consensus view is that over 100 million sequences are needed for analyzing alternative splicing, but due to the high cost, most researchers cannot afford going this deep with their RNA sequencing experiments. Moreover, many medically important genes are not expressed at high levels. Even a deep RNA sequencing experiment cannot generate enough coverage on such genes, making it virtually impossible to measure the genes’ alternative splicing patterns.
In the current study, Xing’s team first drew on large-scale public-domain RNA sequencing data from sources such as the ENCODE Consortium, the international program launched by the National Human Genome Research Institute, to identify all the functional elements in the genome, including those acting at the level of RNA. Using these massive data sets, DARTS trains a deep neural network for predicting changes in alternative splicing. The model incorporates messenger RNA (mRNA) levels of 1,500 RNA binding proteins and 3,000 sequence features.
To allow researchers to use the deep learning model in their own studies, the deep neural network predictions are combined with actual RNA sequencing data generated on specific biological samples using a statistical framework called Bayesian hypothesis testing. Researchers can use this information in their individual labs to better characterize alternative splicing across different biological conditions.
The researchers applied DARTS to lung and prostate cancer cell lines to test its ability to predict splicing patterns in the cells. These cell lines are models for the transition from epithelial to mesenchymal cells — an important process in both embryonic development and cancer metastasis. By leveraging the deep learning predictions, DARTS discovered changes in alternative splicing patterns in numerous genes that escaped detection by conventional computational tools because these genes were expressed at low levels in the cells. The study team then performed experiments to validate these novel predictions. These new discoveries may allow scientists to better identify biomarkers and therapeutic targets of diseases.
“DARTS offers an exciting conceptual framework that we could adapt to other uses,” added Xing. “For example, we might create a version that predicts alternative splicing in specific patient tissues.” This could potentially improve diagnosis of rare diseases from a tissue biopsy, a useful technique for pediatric centers such as CHOP that often evaluate children with puzzling, undiagnosed disorders.
DARTS, Xing concluded, could enable scientists to discover more about the contributions of understudied genes that may not be expressed at high levels, but have important impacts on health and disease. “DARTS offers a new window into the dark matter of the transcriptome,” he said.
DARTS is broadly available to the scientific community at https://github.com/Xinglab/DARTS.
The National Institutes of Health provided funding support for this study (grants R01GM088342, R01GM117624, U01HG007912 and U01CA233074). Disclosure: Xing and study co-author Douglas L. Black of the University of California, Los Angeles, are scientific co-founders of the company Panorama Medicine. Panorama holds a non-exclusive license to the DARTS technology. Xing and co-author Zijun Zhang have filed a provisional patent application for DARTS.
Zijun Zhang et al, “Deep-learning augmented RNA-seq analysis of transcript splicing,” Nature Methods, March 25, 2019.
Learn more about this research in this Cornerstone blog post from CHOP's Research Communications.
A research team at Children’s Hospital of Philadelphia (CHOP) has developed an innovative computational tool offering researchers an efficient method for detecting the different ways RNA is pieced together (spliced) when copied from DNA. Because variations in how RNA is spliced play crucial roles in many diseases, this new analytical tool will provide greater capabilities for discovering disease biomarkers and therapeutic targets, even from RNA-sequencing data sets with modest coverage.
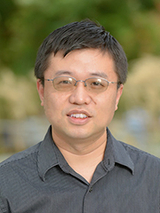
Study leader Yi Xing, PhD, Director of the Center for Computational and Genomic Medicine at CHOP, and first authors and PhD students Zijun Zhang and Zhicheng Pan report on their DARTS framework this week in Nature Methods. DARTS (Deep-learning Augmented RNA-seq analysis of Transcript Splicing) uses deep-learning based predictions to harness the wealth of information available in public datasets of RNA sequencing (RNA-seq), thus allowing for new insights into alternative splicing.
“The conceptual innovation of DARTS is it provides a bridge from big data in the public domain to smaller data sets in focused studies with individual investigators,” said Xing. “DARTS offers the ability to transform massive amounts of public RNA-seq data into a knowledge base, represented as a deep neural network, of how splicing is regulated. Using this computational framework, we can push that into any individual lab. This could be really useful and increase the efficiency of the experiment and enable new discoveries. With just 20 or 30 million RNA-seq reads, you can make educated guesses and inferences on things you were never able to see in the past.”
Xing has a long-standing research focus on alternative splicing — the process by which information in DNA of a single gene is pieced together in different ways to generate different messenger RNA and protein products after gene transcription. Genes each generate an average of 10 or more such products, and sometimes as many as 38,000. Those variations in alternative splicing may cause disease, modify disease risk, or make a disease milder or worse.
Massively parallel RNA sequencing is now the standard technology researchers use to investigate alternative splicing. However, to accurately measure alternative splicing, the RNA sequencing experiments have to go very deep. The consensus view is that over 100 million sequences are needed for analyzing alternative splicing, but due to the high cost, most researchers cannot afford going this deep with their RNA sequencing experiments. Moreover, many medically important genes are not expressed at high levels. Even a deep RNA sequencing experiment cannot generate enough coverage on such genes, making it virtually impossible to measure the genes’ alternative splicing patterns.
In the current study, Xing’s team first drew on large-scale public-domain RNA sequencing data from sources such as the ENCODE Consortium, the international program launched by the National Human Genome Research Institute, to identify all the functional elements in the genome, including those acting at the level of RNA. Using these massive data sets, DARTS trains a deep neural network for predicting changes in alternative splicing. The model incorporates messenger RNA (mRNA) levels of 1,500 RNA binding proteins and 3,000 sequence features.
To allow researchers to use the deep learning model in their own studies, the deep neural network predictions are combined with actual RNA sequencing data generated on specific biological samples using a statistical framework called Bayesian hypothesis testing. Researchers can use this information in their individual labs to better characterize alternative splicing across different biological conditions.
The researchers applied DARTS to lung and prostate cancer cell lines to test its ability to predict splicing patterns in the cells. These cell lines are models for the transition from epithelial to mesenchymal cells — an important process in both embryonic development and cancer metastasis. By leveraging the deep learning predictions, DARTS discovered changes in alternative splicing patterns in numerous genes that escaped detection by conventional computational tools because these genes were expressed at low levels in the cells. The study team then performed experiments to validate these novel predictions. These new discoveries may allow scientists to better identify biomarkers and therapeutic targets of diseases.
“DARTS offers an exciting conceptual framework that we could adapt to other uses,” added Xing. “For example, we might create a version that predicts alternative splicing in specific patient tissues.” This could potentially improve diagnosis of rare diseases from a tissue biopsy, a useful technique for pediatric centers such as CHOP that often evaluate children with puzzling, undiagnosed disorders.
DARTS, Xing concluded, could enable scientists to discover more about the contributions of understudied genes that may not be expressed at high levels, but have important impacts on health and disease. “DARTS offers a new window into the dark matter of the transcriptome,” he said.
DARTS is broadly available to the scientific community at https://github.com/Xinglab/DARTS.
The National Institutes of Health provided funding support for this study (grants R01GM088342, R01GM117624, U01HG007912 and U01CA233074). Disclosure: Xing and study co-author Douglas L. Black of the University of California, Los Angeles, are scientific co-founders of the company Panorama Medicine. Panorama holds a non-exclusive license to the DARTS technology. Xing and co-author Zijun Zhang have filed a provisional patent application for DARTS.
Zijun Zhang et al, “Deep-learning augmented RNA-seq analysis of transcript splicing,” Nature Methods, March 25, 2019.
Learn more about this research in this Cornerstone blog post from CHOP's Research Communications.