Richard D. Wood Jr. Center for Fetal Diagnosis and Treatment
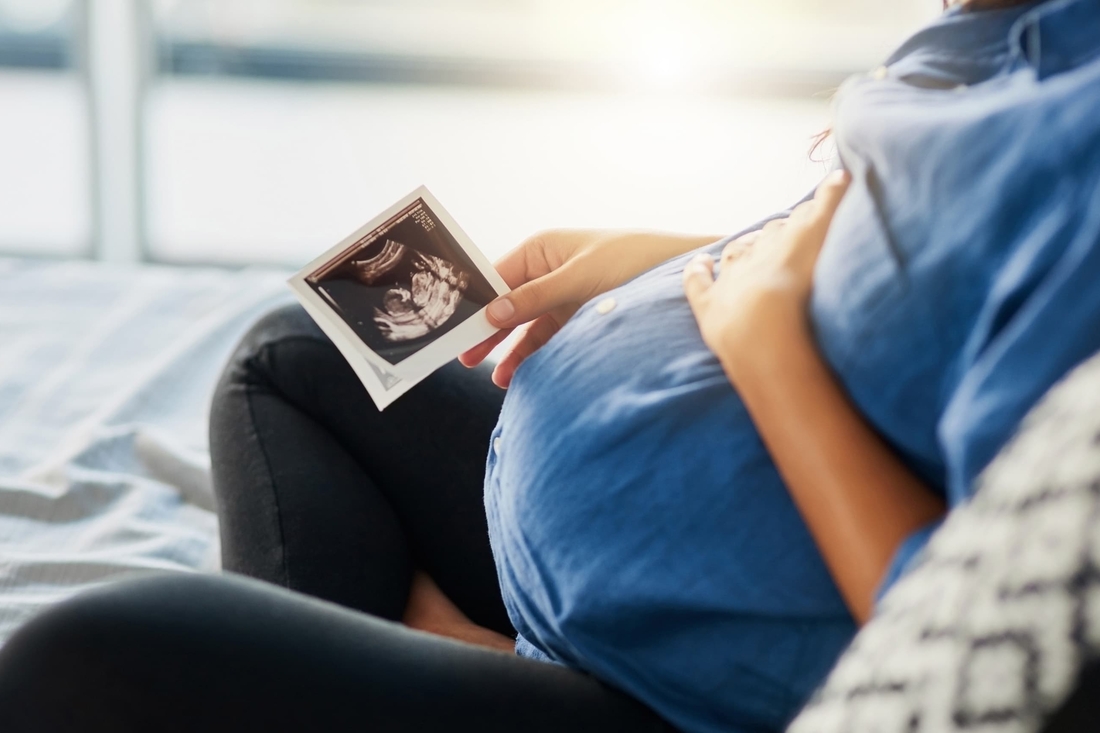
Learning that your unborn baby has a birth defect is overwhelming. It can turn a time of joy into a time of worry. Know that you are not alone. Children’s Hospital of Philadelphia’s Richard D. Wood Jr. Center for Fetal Diagnosis and Treatment is here. We will support you every step of the way, through prenatal diagnosis, pregnancy management, fetal surgery (if necessary), delivery and care after birth. Our medical excellence and decades of experience set us apart. We provide family-centered support services to make what can be a challenging time a little easier for your entire family.
Talk to a fetal therapy nurse coordinator today at 800-468-8376. We will answer as many questions as we can and help make a plan for next steps.
How we serve you
Our fetal center offers highly specialized care for two patients: mother and baby. Here, you have access to the most advanced diagnostic tests, prenatal management, fetal surgery, labor and delivery care, counseling and support services, and expert postnatal care, all in one location. We coordinate all aspects of care and partner closely with your physicians back home.
-
Fetal Heart Program -
Fetal Imaging -
Fetal Surgery -
Fetal Surgery for Sacrococcygeal Teratoma -
Fetal Surgery for Spina Bifida (MMC) -
Garbose Family Special Delivery Unit -
Neonatal Surgical Team -
Newborn/Infant Intensive Care Unit at CHOP -
Prenatal Genetic Counseling -
Psychosocial Support Services -
SDU Newborn Stabilization Team
Conditions we treat
-
Bronchogenic cyst -
Bronchopulmonary sequestration (BPS) -
CCAM/CPAM -
Cleft lip and palate -
Congenital diaphragmatic hernia (CDH) -
Congenital high airway obstruction syndrome (CHAOS) -
Congenital lobar emphysema (CLE) -
Conjoined twins -
Fetal giant neck masses -
Fetal lung lesions -
Gastroschisis -
Generalized arterial calcification of infancy (GACI) -
Hybrid lesions -
Hydrocephalus -
Laryngeal atresia -
Lower urinary tract obstruction (LUTO) -
Omphalocele -
Pleural effusion -
Sacrococcygeal teratoma -
Selective intrauterine growth restriction (sIUGR) -
Skeletal dysplasias -
Small bowel atresia -
Spina bifida -
Twin anemia polycythemia sequence (TAPS) -
Twin reversed arterial perfusion sequence (TRAP) -
Twin-twin transfusion syndrome (TTTS)
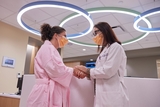
Why choose us
The Wood Center for Fetal Diagnosis and Treatment offers exceptional care for babies with prenatal birth defects. Since 1995, we have helped more than 34,000 families and performed over 2,445 fetal surgeries. We strive for the best outcomes for mother and baby.
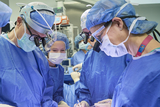
Meet your team
You will receive care from a highly skilled and compassionate team with a global reputation. This team helped shape modern fetal care and continues to improve techniques and treatments.
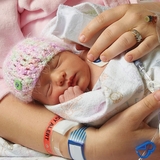
Our research
Every day, we search for new ways to improve outcomes and treat life-threatening conditions before and after birth. Our innovative research projects offer hope for a better future for all children with birth defects.
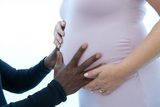
Resources for families
Learning your baby has a birth defect is a life-changing experience. We want you to know that you are not alone. To help you find answers to your questions, we've created this list of educational health resources.
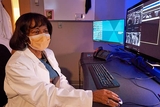
Resources for professionals
Everything you need to support your patient’s health, created and updated by our CHOP community of experts.
Events
Fetal Firesides Virtual CE Lecture Series
Wednesday, May 21, 2025
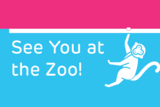
Fetal Family Reunion
Sunday, Jun 01, 2025
What to expect
We understand the anxiety you may be feeling and want you to have the information you need as quickly as possible. Here's what you can expect every step of the way.
Our experience: by the numbers
Our team sees and treats complex anomalies every day. This helps us provide the best possible care for your baby and the best possible experience for you and your family.
Your donation changes lives
Philanthropic donations fuel every breakthrough discovery. With your help, we can continue making advances that will deliver the best possible outcomes for babies with birth defects.
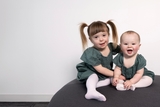